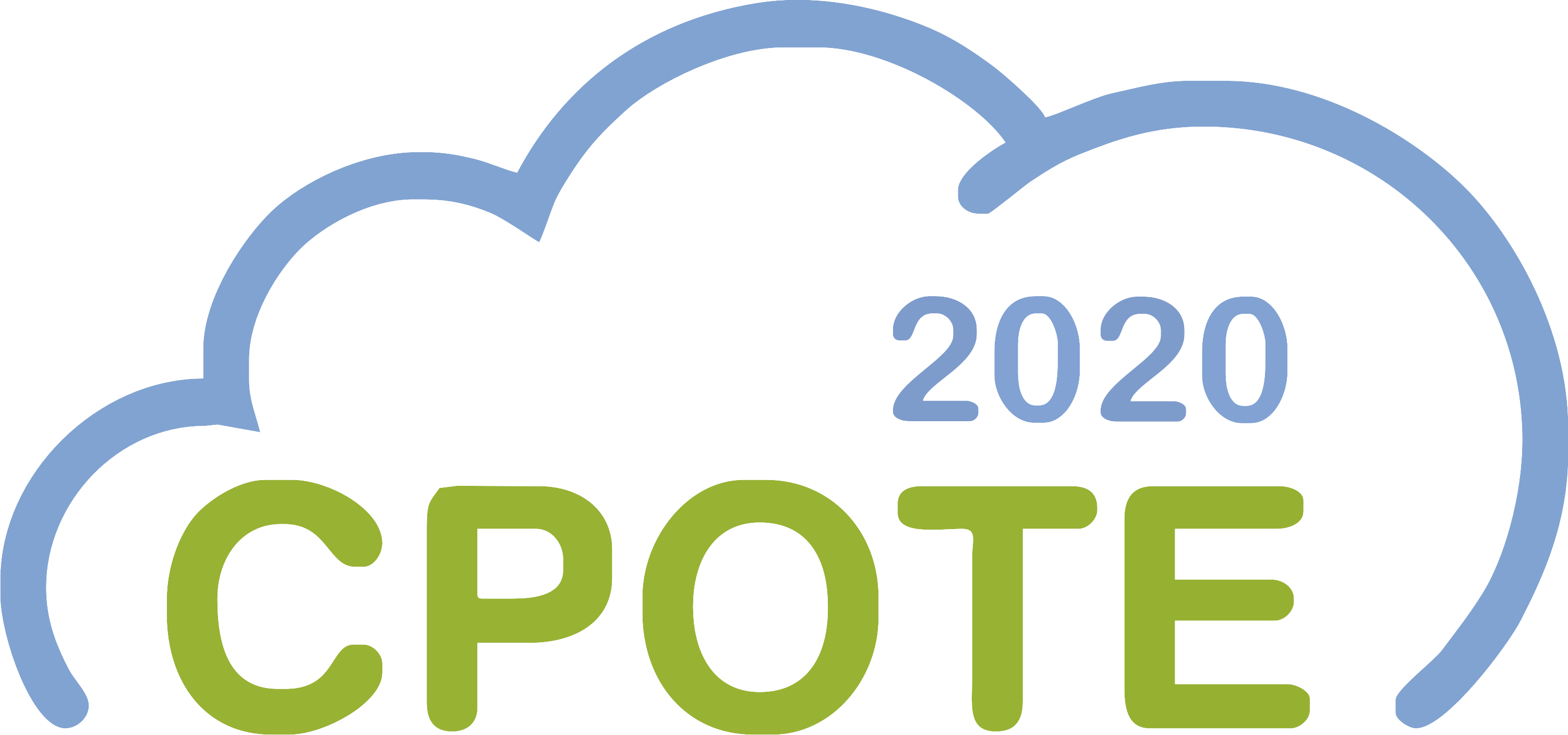
CPOTE2020
6th International Conference on
Contemporary Problems of Thermal Engineering
Online | 21-24 September 2020
6th International Conference on
Contemporary Problems of Thermal Engineering
Online | 21-24 September 2020
Abstract CPOTE2020-1084-A
Book of abstracts draft






Prediction of cost-efficient measures to improve energy access for populations living in energy poverty using modern methods of information technology
Alberto DIAZ DURANA, Technical University Berlin, Germany
This research focuses on developing a methodology using information technology tools and data analysis to identify specific cost-effective measures which could provide households lacking energy access better access to energy. The methodology aims at identifying characteristics of households adopting clean energy technologies. Furthermore, a predictive model to assess the potential for households to move into a higher tier in energy access is proposed. Exploring innovative usage of data to drive social, economic, and environmental impact has become possible through the advances in computational and data sciences. The approach presented in this research includes a combination of an iterative process between statistical analysis and data analysis, known as data science. The data is analyzed using information technology tools for machine learning and data analysis. To this purpose, the energy supply and consumption related to cooking solutions of households in remote rural areas are characterized through a set of attributes that capture key dimensions of energy access, known as the Multi-Tier Framework (MTF). The MTF is a methodology developed by the World Bank to understand what prevents a household from moving to a higher tier of access to affordable, reliable, sustainable, and modern energy in alignment with Sustainable Development Goal 7. This research compares two approaches: (i) classifying the dataset according to a logistic regression based on the MTF classification and (ii) classifying the dataset based on machine learning methodologies to cluster similar households according to a selected dataset. Households with similar features grouped into a common cluster as in (ii) show different tier levels according to the classification described in the MTF as in (i). This difference in the two approaches (i) and (ii) enables the determination of specific sets of variables or features that, when provided to households in the same cluster, but at a lower tier, could enable an improvement in tier and ultimately better access to energy.
Keywords: Energy planning, Energy access, Sustainability, Data analysis, Machine learning
Acknowledgment: I would like to express my deepest gratitude to Professor Tetyana Morozyuk (TU-Berlin, Germany), my thesis supervisor and friend, whose expertise, motivation and encouragement, has granted guidance and support throughout the process of writing my PhD, on which this article is based.